My first professional job during and after college was working at the US Geological Survey as a software engineer and researcher. My job required me to learn about GIS and cartography, as I would do things from writing production systems to researching distributed processing. It gave me an appreciation of cartography and of geospatial data. I especially liked topographic maps as they showed features such as caves and other interesting items on the landscape.
Recently, I had a reason to go back and recreate my mosaics of some Historic USGS Topomaps. I had originally put them into a PostGIS raster database, but over time realized that tools like QGIS and PostGIS raster can be extremely touchy when used together. Even after multiple iterations of trying out various overview levels and constraints, I still had issues with QGIS crashing or performing very slowly. I thought I would share my workflow in taking these maps, mosaicing them, and finally optimizing them for loading into a GIS application such as QGIS. Note that I use Linux and leave how to install the prerequisite software as an exercise for the reader.
As a refresher, the USGS has been scanning in old topographic maps and has made them freely available in GeoPDF format here. These maps are available at various scales and go back to the late 1800s. Looking at them shows the progression of the early days of USGS map making to the more modern maps that served as the basis of the USGS DRG program. As some of these maps are over one-hundred years old, the quality of the maps in the GeoPDF files can vary widely. Some can be hard to make out due to the yellowing of the paper, while others have tears and pieces missing.
Historically, the topographic maps were printed using multiple techniques from offset lithographic printing to Mylar separates. People used to etch these separates over light tables back in the map factory days. Each separate would represent certain parts of the map, such as the black features, green features, and so on. While at the USGS, many of my coworkers still had their old tool kits they used before moving to digital. You can find a PDF here that talks about the separates and how they were printed. This method of printing will actually be important later on in this series when I describe why some maps look a certain way.
Process
There are a few different ways to start out downloading USGS historic maps. My preferred method is to start at the USGS Historic Topomaps site.
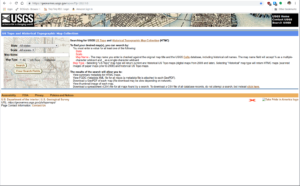
USGS Historic Maps Search
It is not quite as fancy a web interface as the others, but it makes it easier to load the search results into Pandas later to filter and download. For my case, I was working on the state of Virginia, so I selected Virginia with a scale of 250,000 and Historical in the Map Type option. I purposely left Map Name empty and will demonstrate why later.
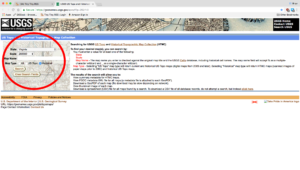
Topo Map Search
Once you click submit, you will see your list of results. They are presented in a grid view with metadata about each map that fits the search criteria. In this example case, there are eighty-nine results for 250K scale historic maps. The reason I selected this version of the search is that you can download the search results in a CSV format by clicking in the upper-right corner of the grid.
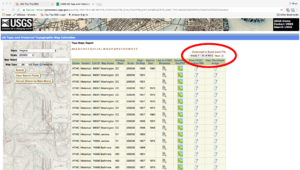
Topo Map Search Results
After clicking Download to Excel (csv) File, your browser will download a file called topomaps.csv. You can open it and see that there is quite a bit of metadata about each map.
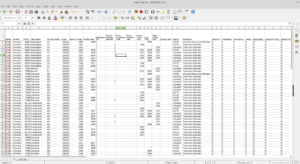
Topo Map CSV Results
If you scroll to the right, you will find the column we are interested in called Download GeoPDF. This column contains the download URL for each file in the search results.
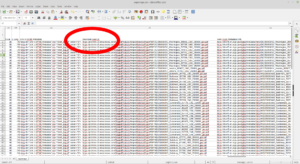
Highlighted CSV Column
For the next step, I rely on Pandas. If you have not heard of it, Pandas is an awesome Python data-analysis library that, among a long list of features, lets you load and manipulate a CSV easily. I usually load it using ipython using the commands in bold below.
bmaddox@sdf1:/mnt/filestore/temp/blog$ ipython3
Python 3.6.6 (default, Sep 12 2018, 18:26:19)
Type "copyright", "credits" or "license" for more information.
IPython 5.5.0 -- An enhanced Interactive Python.
? -> Introduction and overview of IPython's features.
%quickref -> Quick reference.
help -> Python's own help system.
object? -> Details about 'object', use 'object??' for extra details.
In [1]: import pandas as pd
In [2]: csv = pd.read_csv("topomaps.csv")
In [3]: csv
Out[3]:
Series Version Cell ID ... Scan ID GDA Item ID Create Date
0 HTMC Historical 69087 ... 255916 5389860 08/31/2011
1 HTMC Historical 69087 ... 257785 5389864 08/31/2011
2 HTMC Historical 69087 ... 257786 5389866 08/31/2011
3 HTMC Historical 69087 ... 707671 5389876 08/31/2011
4 HTMC Historical 69087 ... 257791 5389874 08/31/2011
5 HTMC Historical 69087 ... 257790 5389872 08/31/2011
6 HTMC Historical 69087 ... 257789 5389870 08/31/2011
7 HTMC Historical 69087 ... 257787 5389868 08/31/2011
.. ... ... ... ... ... ... ...
81 HTMC Historical 74983 ... 189262 5304224 08/08/2011
82 HTMC Historical 74983 ... 189260 5304222 08/08/2011
83 HTMC Historical 74983 ... 707552 5638435 04/23/2012
84 HTMC Historical 74983 ... 707551 5638433 04/23/2012
85 HTMC Historical 68682 ... 254032 5416182 09/06/2011
86 HTMC Historical 68682 ... 254033 5416184 09/06/2011
87 HTMC Historical 68682 ... 701712 5416186 09/06/2011
88 HTMC Historical 68682 ... 701713 5416188 09/06/2011
[89 rows x 56 columns]
In [4]:
As you can see from the above, Pandas loads the CSV in memory along with the column names from the CSV header.
In [6]: csv.columns
Out[6]:
Index(['Series', 'Version', 'Cell ID', 'Map Name', 'Primary State', 'Scale',
'Date On Map', 'Imprint Year', 'Woodland Tint', 'Visual Version Number',
'Photo Inspection Year', 'Photo Revision Year', 'Aerial Photo Year',
'Edit Year', 'Field Check Year', 'Survey Year', 'Datum', 'Projection',
'Advance', 'Preliminary', 'Provisional', 'Interim', 'Planimetric',
'Special Printing', 'Special Map', 'Shaded Relief', 'Orthophoto',
'Pub USGS', 'Pub Army Corps Eng', 'Pub Army Map', 'Pub Forest Serv',
'Pub Military Other', 'Pub Reclamation', 'Pub War Dept',
'Pub Bur Land Mgmt', 'Pub Natl Park Serv', 'Pub Indian Affairs',
'Pub EPA', 'Pub Tenn Valley Auth', 'Pub US Commerce', 'Keywords',
'Map Language', 'Scanner Resolution', 'Cell Name', 'Primary State Name',
'N Lat', 'W Long', 'S Lat', 'E Long', 'Link to HTMC Metadata',
'Download GeoPDF', 'View FGDC Metadata XML', 'View Thumbnail Image',
'Scan ID', 'GDA Item ID', 'Create Date'],
dtype='object')
The column we are interested in is named Download GeoPDF as it contains the URLs to download the files.
In [7]: csv["Download GeoPDF"]
Out[7]:
0 https://prd-tnm.s3.amazonaws.com/StagedProduct...
1 https://prd-tnm.s3.amazonaws.com/StagedProduct...
2 https://prd-tnm.s3.amazonaws.com/StagedProduct...
3 https://prd-tnm.s3.amazonaws.com/StagedProduct...
4 https://prd-tnm.s3.amazonaws.com/StagedProduct...
5 https://prd-tnm.s3.amazonaws.com/StagedProduct...
6 https://prd-tnm.s3.amazonaws.com/StagedProduct...
7 https://prd-tnm.s3.amazonaws.com/StagedProduct...
...
78 https://prd-tnm.s3.amazonaws.com/StagedProduct...
79 https://prd-tnm.s3.amazonaws.com/StagedProduct...
80 https://prd-tnm.s3.amazonaws.com/StagedProduct...
81 https://prd-tnm.s3.amazonaws.com/StagedProduct...
82 https://prd-tnm.s3.amazonaws.com/StagedProduct...
83 https://prd-tnm.s3.amazonaws.com/StagedProduct...
84 https://prd-tnm.s3.amazonaws.com/StagedProduct...
85 https://prd-tnm.s3.amazonaws.com/StagedProduct...
86 https://prd-tnm.s3.amazonaws.com/StagedProduct...
87 https://prd-tnm.s3.amazonaws.com/StagedProduct...
88 https://prd-tnm.s3.amazonaws.com/StagedProduct...
Name: Download GeoPDF, Length: 89, dtype: object
The reason I use Pandas for this step is that it gives me a simple and easy way to extract the URL column to a text file.
In [9]: csv["Download GeoPDF"].to_csv('urls.txt', header=None, index=None)
This gives me a simple text file that has all of the URLs in it.
https://prd-tnm.s3.amazonaws.com/StagedProducts/Maps/HistoricalTopo/PDF/DC/250000/DC_Washington_255916_1989_250000_geo.pdf
https://prd-tnm.s3.amazonaws.com/StagedProducts/Maps/HistoricalTopo/PDF/DC/250000/DC_Washington_257785_1961_250000_geo.pdf
https://prd-tnm.s3.amazonaws.com/StagedProducts/Maps/HistoricalTopo/PDF/DC/250000/DC_Washington_257786_1961_250000_geo.pdf
…
https://prd-tnm.s3.amazonaws.com/StagedProducts/Maps/HistoricalTopo/PDF/WV/250000/WV_Bluefield_254032_1961_250000_geo.pdf
https://prd-tnm.s3.amazonaws.com/StagedProducts/Maps/HistoricalTopo/PDF/WV/250000/WV_Bluefield_254033_1957_250000_geo.pdf
https://prd-tnm.s3.amazonaws.com/StagedProducts/Maps/HistoricalTopo/PDF/WV/250000/WV_Bluefield_701712_1957_250000_geo.pdf
https://prd-tnm.s3.amazonaws.com/StagedProducts/Maps/HistoricalTopo/PDF/WV/250000/WV_Bluefield_701713_1955_250000_geo.pdf
Finally, as there are usually multiple GeoPDF files that cover the same area, I download all of them so that I can go through and pick the best ones for my purposes. I try to find maps that are around the same data, are easily viewable, are not missing sections, and so on. To do this, I use the wget command and use the text file I created as input like so.
bmaddox@sdf1:/mnt/filestore/temp/blog$ wget -i urls.txt
--2018-09-23 13:00:41-- https://prd-tnm.s3.amazonaws.com/StagedProducts/Maps/HistoricalTopo/PDF/DC/250000/DC_Washington_255916_1989_250000_geo.pdf
Resolving prd-tnm.s3.amazonaws.com (prd-tnm.s3.amazonaws.com)... 52.218.194.10
Connecting to prd-tnm.s3.amazonaws.com (prd-tnm.s3.amazonaws.com)|52.218.194.10|:443... connected.
HTTP request sent, awaiting response... 200 OK
Length: 32062085 (31M) [application/pdf]
Saving to: ‘DC_Washington_255916_1989_250000_geo.pdf’
…
…
Eventually wget will download all the files to the same directory as the text file. In the next installment, I will continue my workflow as I produce mosaic state maps using the historic topographic GeoPDFs.